
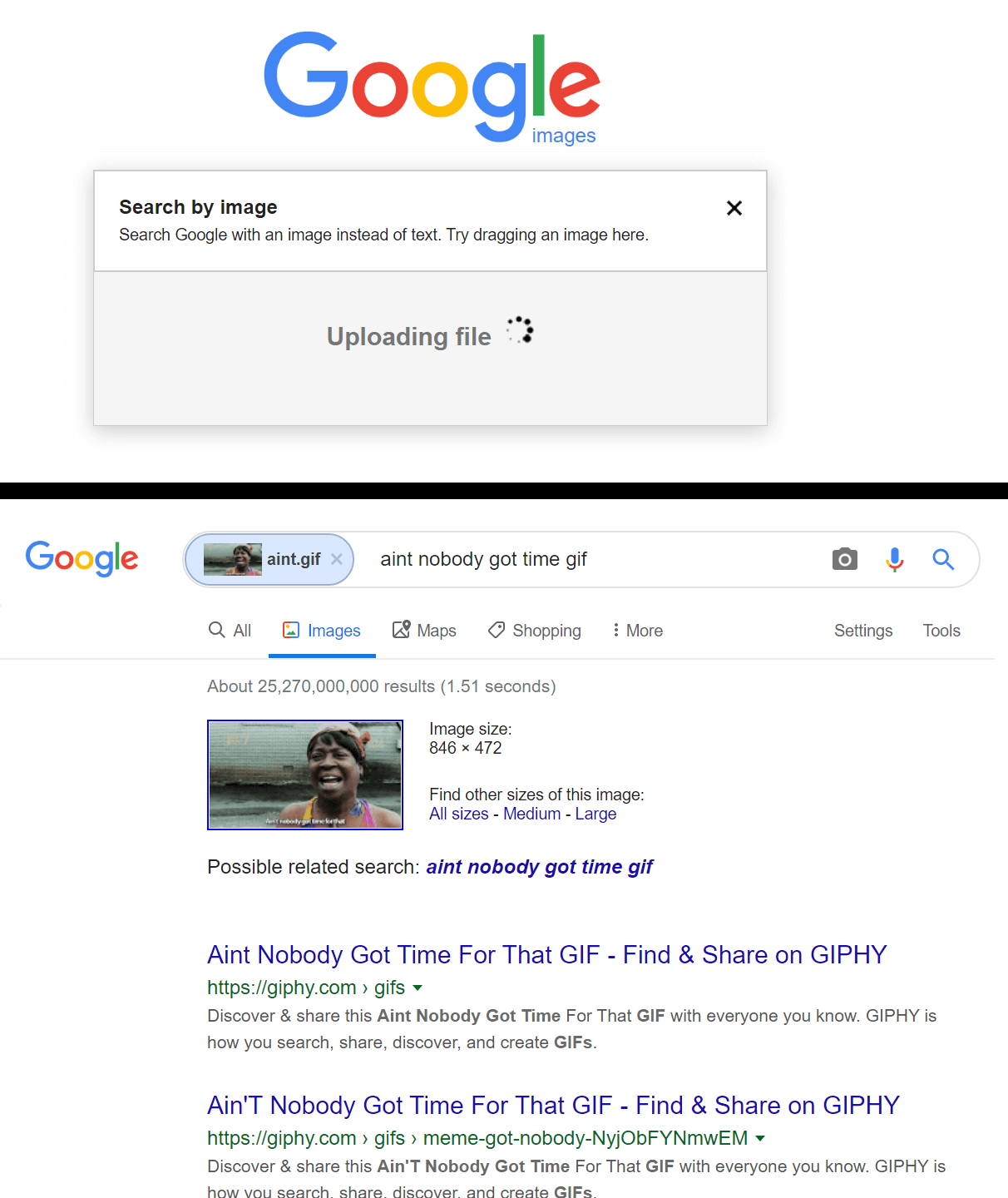
One use case for this approach would be the identification of plagiarism in photographs.Īnother naive approach is to calculate the histogram of RGB values and compare their similarities. By storing the hashes of the patches, duplicates of an image can be found. Although this can help find exact or near-exact images (that might have been cropped), even a slight rotation would result in dissimilarity.

One approach is to compare patches of areas between two images.
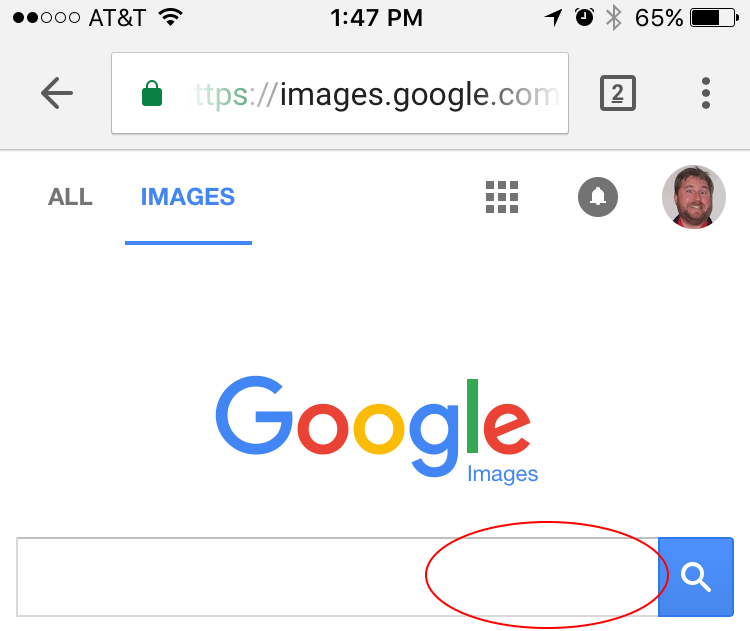
There are several approaches to this problem. The first and foremost question is: given two images, are they similar or not? Making the system more accurate and optimizedĪnalyzing case studies to see how these concepts are used in mainstream products Learning how to scale to large datasets (up to billions of images) Performing feature extraction and similarity search on Caltech101 and Caltech256 datasets The best part is, with the right knowledge, you can build a working replica of many of these products in a few hours. Even face recognition in several security systems uses a similar concept to ascertain the identity of the person.

Sites like TinEye alert photographers on copyright infringement when their photographs are posted without consent on the internet. From discovering visually similar objects on Pinterest to recommending similar songs on Spotify to camera-based product search on Amazon, a similar class of technology under the hood is used. Reverse image search (or as it is more technically known, instance retrieval) enables developers and researchers to build scenarios beyond simple keyword search. After a few minutes of being online, Bob has officially ordered his dream sofa! Taking this more perfect image from the website, he does a few more reverse image searches and finds other websites offering the same sofa at cheaper prices. And searching on the internet with keywords like “white L-shaped,” “modern sofa” gives him thousands of results, but not the one he’s looking for.Īlice hears Bob’s frustration and asks, “Why don’t you try reverse image search?” Bob uploads his images on Google and Bing’s Reverse Image Search and quickly spots a similar-looking image on an online shopping website. So, he takes a few photos from different angles to ask around in local furniture shops, but tough luck: no one knows this particular brand. Asking the office manager doesn’t help either. The brand and model number is not written on the sofa. The bad news is that he doesn’t know where to buy it from. The good news is that he knows what he wants. Then one day, he spots the sofa of his dreams-a unique L-shaped white contemporary sofa in an office reception. He’s flipping endlessly through furniture catalogs and visiting furniture showrooms, but hasn’t yet landed on something he likes. Building a Reverse Image Search Engine: Understanding Embeddingsīob just bought a new home and is looking to fill it up with some fancy modern furniture.
